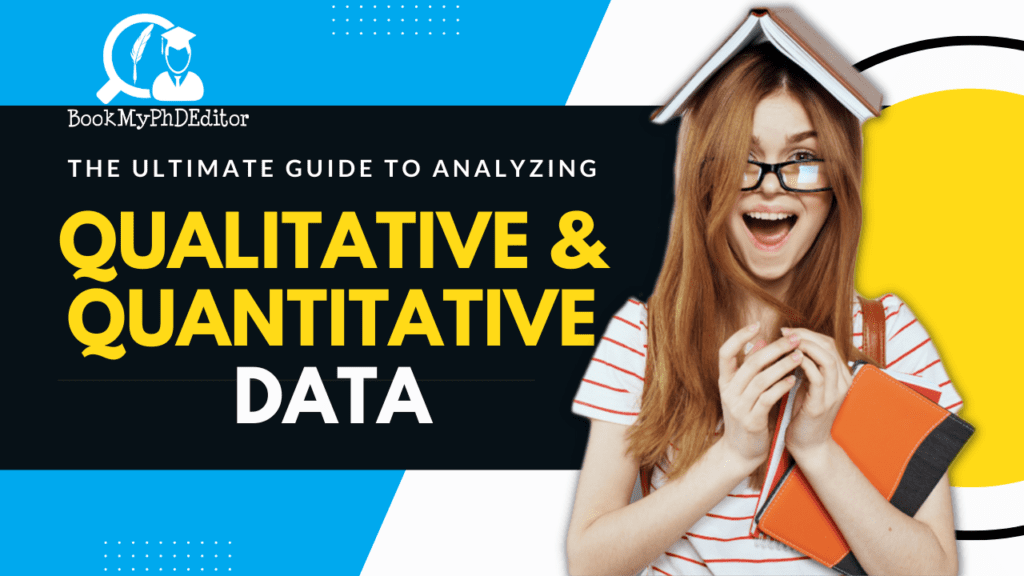
As a Master’s or PhD student, analyzing data is an essential part of your research journey. It is crucial to understand how to analyze both quantitative and qualitative data accurately and effectively to ensure that your research findings are reliable and valid. In this article, we will provide you with an ultimate guide to analyzing quantitative and qualitative data for your Master’s dissertation or PhD thesis.
1. Introduction
Before we delve into the methods of analyzing quantitative and qualitative data, let us first define what these terms mean.
Quantitative data refers to data that can be measured and expressed numerically. Examples include age, income, or number of sales. In contrast, qualitative data refers to data that is descriptive and cannot be measured numerically. Examples include observations, opinions, and interviews.
Analyzing quantitative and qualitative data is crucial in your research journey as it helps you to understand your research findings better. Analyzing data can also help to identify patterns, relationships, and trends in your data, which can help you to draw conclusions.
Let us now walk you through the methods of analyzing quantitative and qualitative data in detail. We will also highlight the importance of data preparation and the use of software tools for data analysis.
2. HOW TO ANALYZE QUANTITATIVE DATA
2.1 DATA PREPARATION
Before analyzing quantitative data, it is essential to prepare the data to ensure that it is reliable and valid. Data preparation involves several steps, including data validation, data editing, and data coding.
Data validation is a crucial step in the process of analyzing quantitative data as it helps to ensure the accuracy and reliability of the data. To validate data, one approach is to use a four-step model consisting of fraud, screening, procedure, and completeness.
The first step, fraud, involves checking for any false or misleading information in the data. This could include checking for duplicate responses or inconsistent answers. The next step, screening, involves reviewing the data for any errors or omissions. For instance, in the case of survey data, one could check for incomplete surveys or missing responses.
The third step, procedure, involves ensuring that the data was collected using appropriate methods and that the data collection process was conducted consistently. This could involve checking the sampling method, the survey questions, and the data collection procedures to ensure that they were standardized and consistent.
Finally, the completeness step involves verifying that all the required data has been collected and that there are no gaps in the data. This could involve checking for any missing data or incomplete responses.
By following this four-step model for data validation, researchers can ensure that their data is accurate and reliable, which is essential for conducting meaningful data analysis.
Data editing involves identifying and correcting errors in the data. For example, if you find an outlier in your data, you may need to remove it or adjust it to ensure that it does not skew your results.
Data coding involves assigning numerical values to data to make it easier to analyze. For example, if you collected data on respondents’ levels of education, you could assign numerical values to different education levels, such as high school (1), college (2), or graduate school (3).
It is crucial to perform these steps to ensure that the data is accurate and reliable.
2.2 METHODS OF QUANTITATIVE DATA ANALYSIS
After preparing the data, you can proceed to analyze it. There are two main types of quantitative data analysis: descriptive and inferential statistics.
Descriptive statistics are used to summarize and describe the data. Examples of descriptive statistics include mean, median, mode, percentage, frequency, and range. For example, if you collected data on the number of hours worked by employees in a company, you could calculate the mean or median to describe the typical number of hours worked by employees.
Inferential statistics are used to make inferences or predictions about the population based on the sample data. Examples of inferential statistics include correlation, regression, and analysis of variance. For example, if you collected data on the relationship between customer satisfaction and sales, you could use regression analysis to determine whether there is a significant relationship between the two variables.
There are several software tools available for analyzing quantitative data, such as SPSS, Excel, and Stata. These tools can help you to perform complex statistical analyses and produce accurate and reliable results.
It is important to interpret and present the results of your quantitative data analysis clearly and concisely. Your research findings should be presented in a way that is easy to understand and relevant to your research question.
3. HOW TO ANALYZE QUALITATIVE DATA
Qualitative data analysis is a process of identifying patterns, themes, and meanings within the data. It is an exploratory approach that involves the interpretation and analysis of non-numerical data. Qualitative data can come in various forms, such as interviews, focus groups, observations, and document analysis. Here are the steps involved in analyzing qualitative data.
3.1 Data Preparation and Basic Qualitative Data Analysis
Data transcription: The first step in analyzing qualitative data is transcription, which involves converting recorded data into a written format. This process helps to organize the data and make it easier to analyze. Transcription can be done manually or with the help of software tools. A good transcription should include all the details from the data, such as verbal cues, nonverbal expressions, and tone of voice.
Data reduction: Once the data has been transcribed, the next step is data reduction, which involves summarizing the data into manageable categories or themes. This process helps to identify patterns and themes within the data. There are various techniques for data reduction, such as content analysis, discourse analysis, thematic analysis, and narrative analysis.
Data display: After data reduction, the data can be displayed in various formats such as tables, matrices, and graphs. Data display helps to organize the data and make it easier to understand.
3.2 Methods of Qualitative Data Analysis
Content analysis: Content analysis is a technique used to analyze text-based data such as interviews, focus groups, and open-ended survey questions. The aim is to identify patterns and themes within the data. The process involves coding the data into categories or themes and then interpreting the findings.
Discourse analysis: Discourse analysis is a technique used to analyze language-based data such as interviews, focus groups, and written documents. The aim is to understand how language is used to convey meaning and how it shapes social reality. The process involves analyzing the language used and identifying the underlying meanings and assumptions.
Thematic analysis: Thematic analysis is a technique used to identify patterns and themes within qualitative data. It involves coding the data into categories or themes and then interpreting the findings. The aim is to identify patterns and themes within the data.
Narrative analysis: Narrative analysis is a technique used to analyze stories or accounts provided by participants. The aim is to understand how people construct their experiences and how they make sense of their lives. The process involves identifying the key elements of the story and analyzing the underlying meanings.
Grounded theory: Grounded theory is a technique used to develop theories based on qualitative data. The aim is to develop a theory that is grounded in the data rather than starting with a preconceived hypothesis. The process involves analyzing the data and identifying key concepts, which are then used to develop a theory.
Importance of Software Tools in Qualitative Data Analysis
There are various software tools available to aid in the analysis of qualitative data, such as NVivo, MAXQDA, and Atlas.ti. These tools can help organize the data, identify patterns and themes, and facilitate data display. The use of software tools can also save time and increase the accuracy of the analysis.
Interpreting and Presenting the Results
Interpreting and presenting the results of qualitative data analysis is important to communicate the findings effectively. The results can be presented in various formats such as tables, charts, and graphs. The presentation should be clear, concise, and easy to understand. It is also important to provide a clear explanation of the findings and how they relate to the research questions.
In conclusion, analyzing both quantitative and qualitative data is crucial for any master’s dissertation or PhD thesis. The methods outlined in this guide can serve as a starting point for students looking to analyze their data effectively.
Data analysis can be a daunting task, and it is important to have a clear understanding of the methods and techniques involved. Seeking the assistance of professional academic editors and data analysts can help ensure that your research is of the highest quality.
At Book My PhD Editor, we offer a wide range of academic services, including academic editing, proofreading, and data analysis, to help PhD and Master-level students produce top-quality academic drafts. Contact us today at info@bookmyphdeditor.com to learn more about how we can help you with your research.